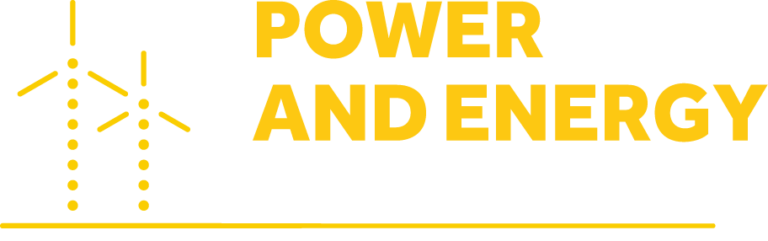
towards the future of the energy systems
About the Webinars
INESC TEC, through its Centre for Power and Energy Systems and the Energy cluster, has launched the Power and Energy Webinar Series initiative. In each webinar, a researcher from INESC TEC will present and discuss ideas, expected outcomes, or results regarding the energy systems of the future. In some webinars, external speakers will be invited. The expected duration of the webinar is between 45 minutes and 1 hour, depending on the format. Discussion between the speakers and the participants will be held in the last part of each webinar.
Next Webinar
Data markets and sharing in the energy sector
May 15 • 14:00 (GMT +1) • 15:00 (CET)
Smart meter data sharing in energy systems
Yi Wang , Assistant Professor of the DEEE, The University of Hong Kong
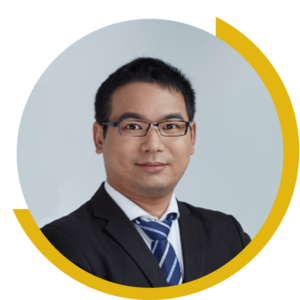
With the digitalization of the power and energy systems, various data will be collected by widely installed sensors. Data-driven technologies have been adopted to transform massive smart meter data into knowledge, providing deeper insights into the past, better understandings of the future, and practical advice on possible decisions. However, the barrier of data sharing across sectors resists the application of these data-driven technologies. To overcome this barrier, the construction of a data market is a promising solution that would allow for the well exploitation of data value to benefit data owners and break down data barriers. This talk introduces the concept of a data market, including the attributes of data as a commodity, the structure of the data market, strategies for valuing data, and the settlement process within the data market. Additionally, this presentation will highlight our attempt to construct an end-to-end data valuation framework approach by integrating forecasting and decision processes in multi-energy systems. The proposed valuation approach effectively extracts the inherent data value, and all sectors can benefit from data sharing by improving forecasting accuracy or receiving economic compensation.
Algorithmic Solutions for Data and Analytics Markets
Carla Gonçalves, Researcher at INESC TEC
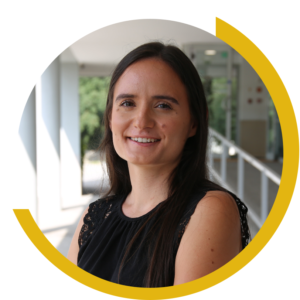
Cooperation between data owners may lead to an improvement in forecast accuracy – for instance by benefiting from spatial-temporal dependencies in geographically distributed time series. However, for data owners to perform collaborative forecasting models, two important properties are required: that data privacy is ensured during the collaboration, and that data owners are compensated for the data they contribute (data markets). The focus of this presentation is on the development of algorithmic solutions for data and analytics markets. Two incentive schemes will be considered: i) monetary incentives, where data owners accept to share their data because they are monetarily compensated if their data is relevant for solving analytics/optimization tasks and paid in case data from the others is relevant to their own tasks; ii) non-monetary incentives, where there is no money involved and data owners agree to share and receive data with approximately the same value.
I want to participate
PRIVACY POLICY:
The personal data collected by answering the registration form will be used by INESC TEC, as a data controller, for the sole purpose of registration and management of the event. The data will be eliminated within 15 days after the event.
Additional information
You can contact our DPO in case you have any questions related to the processing of personal data or to the exercise of your rights through the email dpo@inesctec.pt.
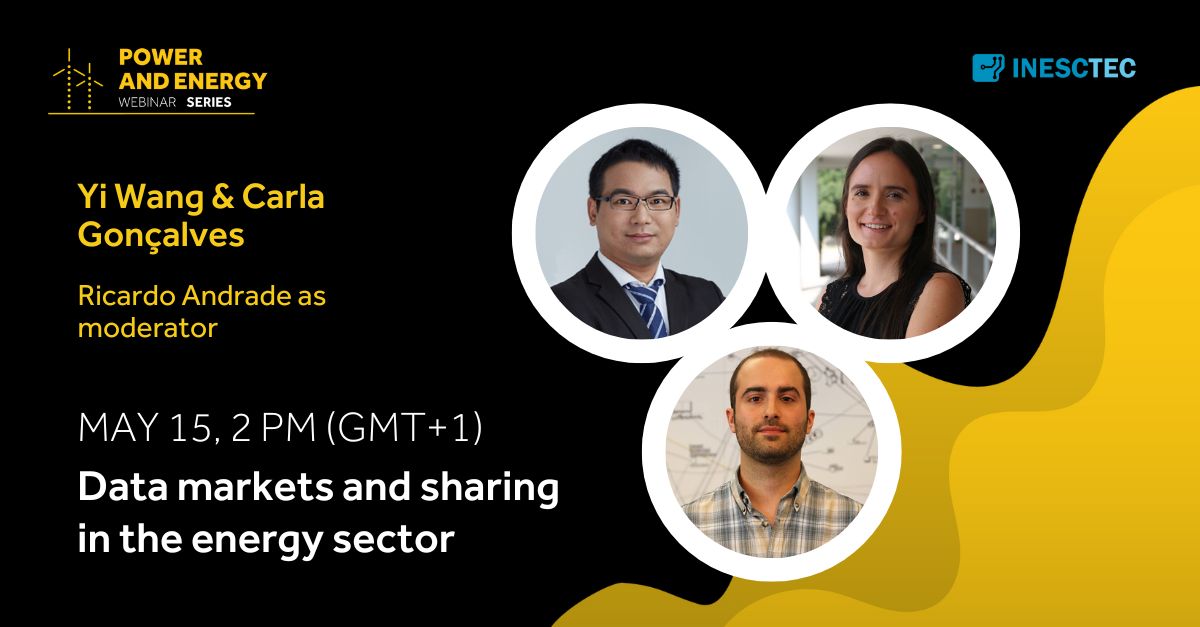
About the speakers & moderator:
Yi Wang, Assistant Professor of the DEEE, The University of Hong Kong
Yi Wang is currently an Assistant Professor of the Department of Electrical and Electronic Engineering, The University of Hong Kong. He received his bachelor’s degree in electrical engineering from Huazhong University of Science and Technology (HUST) in June 2014, and Ph.D. degree in electrical engineering from Tsinghua University (supervised by Prof. Chongqing Kang) in Jan. 2019. From March 2017 to April 2018, he was an exchange student researcher at the University of Washington. From Feb. 2019 to Aug. 2021, he served as a Postdoctoral Researcher in the Power Systems Laboratory, ETH Zurich. Yi Wang’s research interests include Data analytics in the smart grid, energy forecasting, multi-energy systems, Internet-of-things, cyber-physical-social energy systems. Yi Wang currently serves as the secretary of IEEE Customer Systems & Smart Buildings Subcommittee, the secretary of IEEE PES Working Group on Energy Forecasting and Analytics, and the secretary of CIGRE Working Group on Application of 5G Technology to Smart Grids. He also serves as the Associate Editor for IEEE Transactions on Smart Grid, IEEE Systems Journal, and IET Renewable Power Generation.
Â
Carla Gonçalves, Researcher at INESC TEC
Carla Gonçalves is a researcher at the Centre for Power and Energy Systems in INESC TEC. In 2021, she obtained a Ph.D. in Applied Mathematics from the Faculty of Sciences of the University of Porto (FCUP). From 2015 to 2019, she was involved in a wide range of energy forecast consulting collaborations between INESC TEC and the industry: REN (Portugal), EDP Renewables (Spain), RTE (France), and EDP Gestão da Produção de Energia (Portugal). Until 2022, she was part of the H2020 Smart4RES project, and since 2023, she has been taking part in the European ENERSHARE and GREEN.DAT.AI projects. Her research has been focused on probabilistic and collaborative forecasting methods, with a particular emphasis on renewable energies and data-sharing incentive mechanisms.
Â
Ricardo Andrade, Researcher at INESC TEC
Ricardo was born in 1992 in Sta. Maria da Feira, Portugal, received the M.S. degree from the Faculty of Engineering of the University of Porto, Portugal (FEUP) in 2016 in Electrical and Computer Engineering. His main interests are data analysis/visualization and machine learning concepts applied to renewable energy and electricity prices forecasting.
Past Editions
To access the videos you need attend the webinars or contact us directly to energywebinars@inesctec.pt
Webinar 20
January 17, 2024)
Webinar 19
(December 7, 2023)
Webinar 18
(June 19, 2023)
Webinar 17
(January 31, 2023)
Webinar 16
(November 24, 2022)
Webinar 15
(October 27, 2022)
Webinar 14
(September 29, 2022)
Webinar 13
(July 14, 2022)
Webinar 12
(January 17, 2022)
Webinar 11
(December 16, 2021)
Webinar 10
(November 17, 2021)
Webinar 9
(October 27, 2021)
Webinar 8
(July 7, 2021)
Webinar 7
(May 21, 2021)
Webinar 6
(March 26, 2021)
Webinar 5
(February 1, 2021)
Webinar 4
(November 27, 2020)
Webinar 3
(October 26, 2020)
Webinar 2
(September 14, 2020)
Webinar 1
(July 23, 2020)
Contact us
Send us an e-mail and we will contact you as soon as possible
- energywebinars@inesctec.pt
PRIVACY POLICY: The webinars will be entirely recorded. The videos/images captured will only be used for promotional and informational purposes. Therefore, the Power and Energy Webinar Series can use your picture/video in publications, such as on websites, newsletters or social media platforms, among others. If you do not want your image to be stored for dissemination purposes, please let the event organisation know.